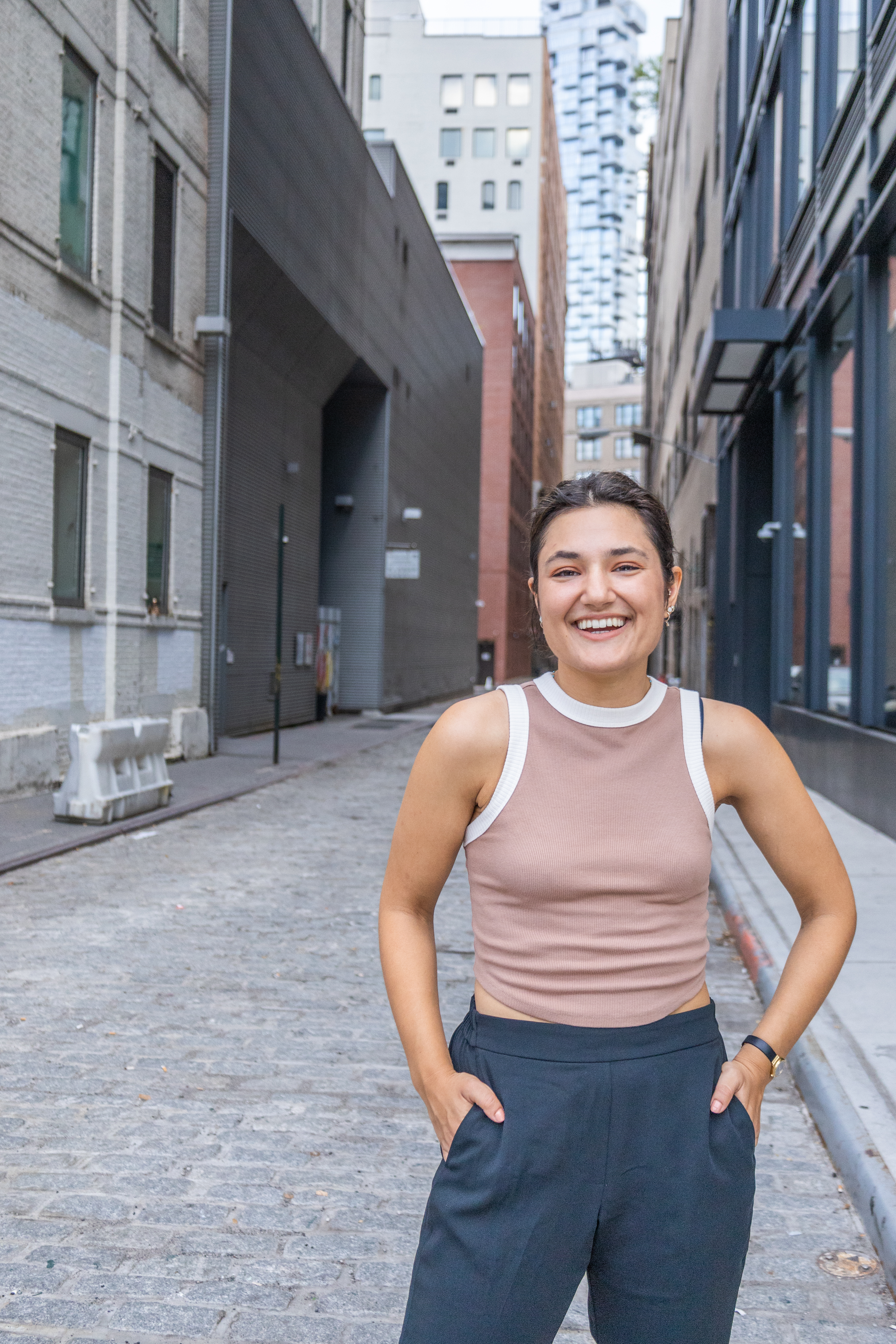
Hi, I'm Julie. I'm an NYC-based machine learning engineer working on recommendation systems. I am also a TA for AP Computer Science Principles at Pelham Preparatory Academy, and I sit on the board of CCARC in Connecticut. I'm currently studying for my Master's Degree at NYU, focusing on the ethical and responsible development of machine learning and artificial intelligence.
I've written and contributed to a few papers as I've been working on my master's degree.
Final project for fair and ethical ML in fall of 2024
Abstract
Machine learning models, despite their potential benefits, can perpetuate and amplify existing societal biases present in training data. This raises concerns about fairness, particularly when these models are deployed in high-stakes applications, such as credit scoring, hiring, and criminal justice. A key challenge in mitigating bias is the incompatibility among different fairness definitions. This paper attempts to address this challenge by introducing a novel framework to balance multiple, potentially incompatible fairness definitions using composite adversarial training. We find that there is some promise to this approach, though more work needs to be done to ensure the stability of the system.
Proposed in June 2024 for NYU Gallatin's graduate program
Summary
Early methodologies for bias investigation would frequently involve pre-defining a set of groups for some protected attribute (like race or gender) and requiring parity of some fairness statistic across all of these groups. While this may appear to enforce fairness for all groups within the protected class, this methodology does not protect the subgroups of these groups. For example, if fairness is enforced by parity for all genders and for all age ranges, parity may not hold when looking at a group of young women and a group of young men. Given this, it is not enough to simply enforce fairness at the group level. Fairness must also be maintained for the exponentially large set of subgroups within a population.
Working group member with Partnership on AI from January 2023 through April of 2024
Executive Summary
The Guidelines aim to provide AI developers, teams within technology companies, and other data practitioners with guidance on collecting and using demographic data for fairness assessments to advance the needs of data subjects and communities, particularly those most at risk of harm from algorithmic systems. Central to this resource is the concept of data justice, which asserts that people have a right to choose if, when, how, and to what ends they are represented in a dataset.
Final project for machine learning in fall of 2023.
Abstract
Garbage in, garbage out is an old colloquialism in computer science, and it is never more true than in machine learning. As society continues to ask machine learning systems to make increasingly high impact decisions, it becomes increasingly im- perative to recognize the importance of unbiased representation in the datasets used to train and test models. This work demonstrates how easily a biased perspective can become the basis for predictions in a classification model, and offers a simple method to mitigate this bias.
Final paper for sociology in fall of 2023.
Introduction
Social media’s impact on human society is undeniable. We use it compulsively; it’s frequently characterized colloquially and, in some cases, formally, as an addiction. More and more of our interactions take place through various social media platforms, shaping the ways we view and navigate the world. As a result, the physical spaces that have been used as forums to share ideas—public realms—have receded, even disappearing altogether in some cases, taking with them the sense of shared reality among people. This lack of connection and understanding damages our ability to connect to one another and brings to light the very real consequences of this loss.